See the Living Loops
Some control comes for free
Marco Giancotti,
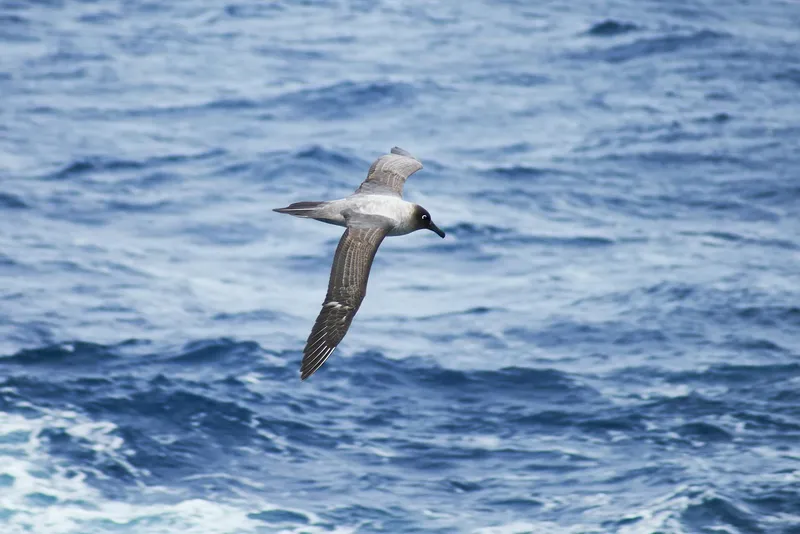
Marco Giancotti,
Cover image:
Photo by Tony Wright, Pexels
The Muddy Middle Ground
In a recent Plankton Valhalla essay, I made the bold claim that recursion—things influencing their own future through their behavior—is at the core of everything we can know and reason about. "Feedback," I wrote, "makes recursion possible, and recursion is what makes the unlikely likely." That unlikely-made-likely thing that a feedback loop produces, whatever it is, I call a Water Lily. The reason I wrote a long essay, full of examples, to emphasize that point, and the reason I coined that strange term, is that I believe it is a very important framing to have even in our daily lives. Feedback loops aren't scientific curiosities or rare phenomena that we might read about occasionally and then forget: they are everywhere, and we should all be intimately familiar with how they work.
I'll repeat the definition of a Water Lily once more, because it will come up frequently in what follows.
A Water Lily is the particular state, out of many possibilities, that a system tends toward as the result of a feedback loop.
In order to understand these supposedly ubiquitous loops, we need to see them, and seeing them can be very tricky at times. Some are easy to spot—we often talk of "downward spirals of depression" and "virtuous cycles of economic growth", for example—but what about all the others?
In the essay, I provided plenty of examples of non-living, natural feedback like smooth pebbles and "tidy stars". These are fascinating and important, but they tend to be less relevant at the day-to-day level. I also explained the kind of feedback that is possible at the other extreme of the spectrum, something called "control mechanisms". Here the feedback loop is clearly a repeating sequence of observing, measuring the discrepancy from a goal, and acting to remove the discrepancy. Control, in this sense, is extensively studied in various fields of knowledge, from robotics to biology to sociology, and much has already been written about it.
But it is especially the murky middle ground between those two extremes that can create confusion: what about living and engineered feedback loops that are not "control" in the proper sense of the term? What about goal-less processes that nonetheless seem to be achieving useful outcomes? That is what I will try to illuminate below.
I think that most people's intuition is that a brain is necessary to achieve anything useful and difficult in this harsh world. And if not a full-blown brain, surely an organism needs at least a nervous system to sense the world and make decisions about what to do about it, or so the thinking goes.
Not so, because—to expand on a point already touched upon in the essay—feedback is much more fundamental than that.
All living things are powered by some kind of "embedded prophesy devices", i.e. internal models of the world. To remain stable or achieve an unlikely state (a Water Lily), an organism must be able to predict the future to some extent and choose its course of action based on those predictions. But prediction is impossible without some form of input from the environment. So the question becomes what that feedback, and those prophecies, could possibly look like when no brain and no nerve-based senses are involved. Some examples will bring us closer to an answer.
Always Start with the Dead Ones
When talking about feedback, the easiest place to begin is usually non-living, human-made stuff.
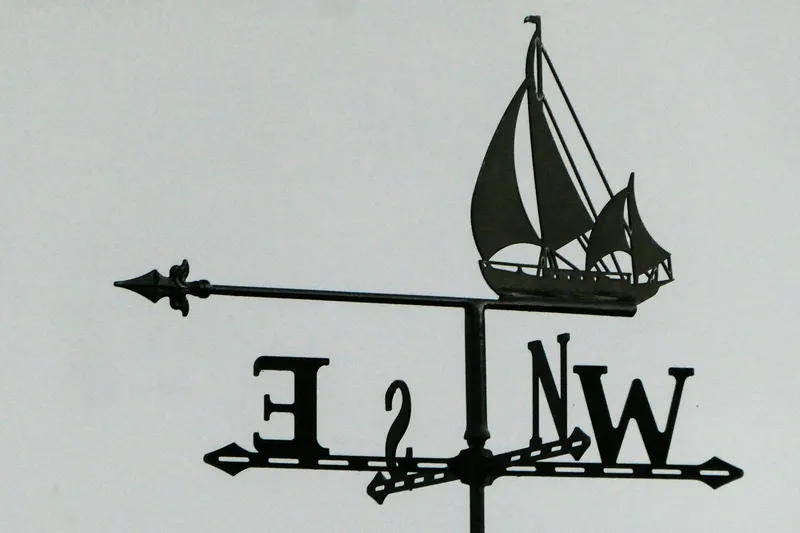
Consider, for example, the weather vane. It is essentially a flat surface attached to a rotating pole. When the wind is strong enough, the pressure from the incoming air creates a force that turns the weather vane, with a strength proportional to its current angle relative to the airflow. This is clearly a feedback loop, its Water Lily being the state of aligning with the wind's direction. Since this Water Lily was explicitly chosen by a human designer, it belongs to the category of Water Lilies known as "goals".
Notice how this simple system, devoid of brains, sensors, or any kind of wire, can correct the discrepancy between its Water Lily state and the current state.
Other examples include the moving bicycle's tendency to remain vertical on its own and the paper airplane's ability (when well-folded) to go on gliding horizontally and without flipping until it touches the ground. These sensor-less feedback loops are called passive control in engineering because there is no "conscious", active deliberation on the best course of action: they just seem to "know" what to do automatically.
Passive control mechanisms are the unsung heroes of so much modern (and ancient) technology, humbly supporting fancier cybernetic advances while being mostly overshadowed by them. With some practice, you can learn to spot them literally everywhere. They were the first "control" processes to be invented (see James Watt's famous governor in steam engines). They are in every analog mechanism (like car dampers and roly-poly dolls), but also in those that are actively controlled, which we'll return to later.
Take a moment to consider just how unlikely all those outcomes would be otherwise—the bicycle staying vertical, the weather vane's arrow pointing at the "N" sign precisely when the wind comes from the north, the airplane not randomly tumbling like a dead leaf. It is through these recurring cycles of gradual and unthinking "error-correction" that they occur reliably enough for everyday use.
Of Course, Evolution Was there First
Moving on to living things, the most obvious examples are plants, which are known to adapt and react to their environment even without neurons or central processing units. But I have already described how there is no script in the life of a Water Lily before, so here are other examples.
The albatross, the greatest voyager of the skies, can soar for days at a time without expending much energy at all: they have tendons capable of locking the wings into a spread-open position like umbrellas, and they stay open even against the constant pushing and tugging from the wind currents. Once extended like that, the wings also participate in other passive stabilizing feedback loops, similar to those that keep a paper plane aloft, so the bird doesn't have to worry too much about sudden pressure dips or turbulence.
Evolution has produced many other recursion-based processes. Scientists have found, for example, that trout are able to swim upstream even while dead, purely because of the hydrodynamic properties of their bodies.
Sea sponges, among the very few animals without a nervous system to speak of, can still detect when there is too much silt in their pores and activate a sort of slow-motion sneeze to expel it. This happens because silt triggers additional production of calcium in their surface cells, which in turn induce calcium production in nearby cells, and so on in a cascade that makes them all contract in turn over the course of half an hour, freeing the sponge of the unwanted materials.
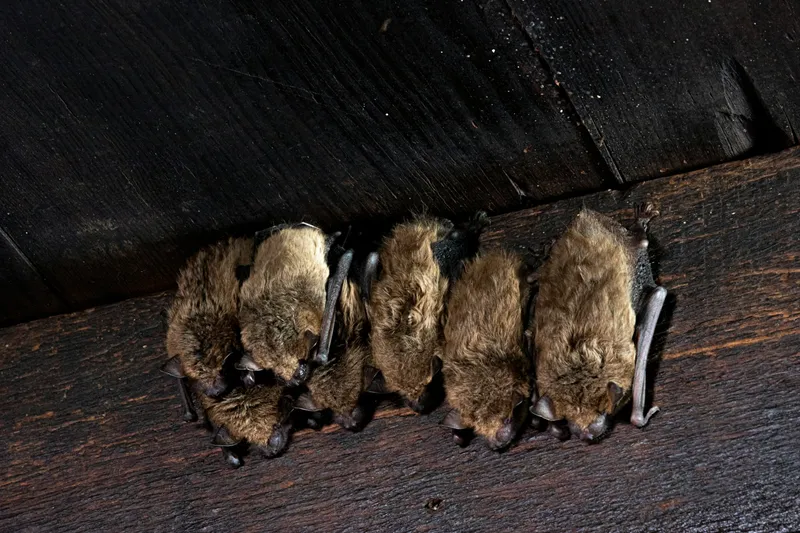
Likewise, the human skin (to pick one example out of many in the human body) adapts to the outside conditions independently from any nervous activity. Soon after I started playing badminton a couple of years ago, a callus formed precisely in the spot of my right palm where the grip of the racket is tightest. The stress applied to those skin cells by the racket encouraged them to produce much more keratin, a tough, fibrous protein that accumulates to form what we call a callus. A bit more keratin allows me to play badminton a little longer without rupturing my skin, which in turn induces more stress and more keratin production, and so on until the callus is thick enough to completely shield my skin cells from the wear and tear of my (amateurish) smashes.
Here, again, we see the same pattern of unlikely things—bats and soaring birds not crashing down at the first vibration, clean sea sponges, skin stronger in all the right spots—happening regularly and reliably thanks to self-enforcing mechanisms, completely independent of active control or commands from specialized "thinking organs". These are the Water Lilies that give shape and stability to the most fundamental of living functions.
The Middle of the Middle of the Way
You may not be surprised to learn that the boundary between "passive" and "active" control is very fuzzy. Many technological and biological processes straddle the two approaches. A modern aircraft in flight, for example, is stabilized both by its inherent aerodynamic shape—technically called positive static stability—and by the complicated operation of many sensors and flaps—i.e., traditional control.
Or think about how urination works: the conscious "I need to go" impulse arises in your brain after the bladder is filled beyond a certain threshold, before which the control of the urethra valves is automatic and "locked" almost like the albatross' wings. This allows you to forget about that passive feedback loop most of the time, while giving you almost full control when the bladder is filled. It's a clear handover from an evolutionarily tuned Water Lily (you not wetting your pants) to a conscious goal ("I will do it once I get to the W.C."): the "mechanical" parts are the same in both phases, but they're driven by different "circuits" and computational systems.
An E. coli bacterium is another interesting example because it showcases a very common kind of cellular feedback loop positioned halfway between the works-without-batteries approach of a locking tendon and the sensor-actuator approach of a person threading a needle.
E. coli does have something akin to elementary "sensors", similar to the eyes or ears of an animal, despite not having any nerves (it is a single cell, after all), using a simple but genius-level protein-based mechanism. This excellent video by W. W. Norton & Company explains it more clearly than I can hope to achieve in words, but here is the gist. E. coli can rotate its flagella (hair-like motors) in two modes, a random tumbling motion and a straight-line motion. In stable conditions, the switching between these two modes is random, leading to an aimless movement going nowhere in particular. But if, during one of its straight lunges, the concentration of nutrients happens to increase, this will trigger a chain reaction in the movement and production of molecules inside the bacterium, which in turn inhibits the flagella from switching back to the tumbling mode.
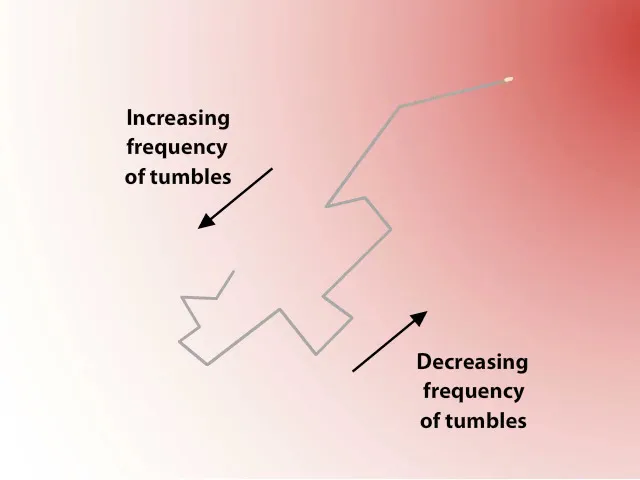
This process means that the cell is more likely to keep swimming straight ahead if it happens to be moving toward a source of nutrients, but will otherwise change direction frequently. This has a clear effect of feedback-induced skewing of probabilities: even though the cell always changes direction randomly, and it doesn't have any idea what it's doing, the probability of it getting closer to its source of sustenance (its Water Lily) is higher than that of moving away.
This type of feedback is simple enough to recognize as a slightly fancier version of a weather vane, passively turning in the wind. At the same time, it involves a few intermediate steps to function, in the form of proteins within it being activated and moving molecules around. This is reminiscent of how long chains of nerves carry information around through electrochemical means.
Here, then, is the key insight: there is no sharp division between "passive" and "active" control, but there is a gradation in how direct or indirect the error-correcting mechanism is—how many steps it involves.
The common theme we see in all these examples is that the model of how the world works is built into the structure of those systems, and it operates based on basic physical laws. Contrast this with brain- and computer-based control, where there is this thing we call "information" being shuttled around by axons or integrated circuits, stored somewhere in a rather abstract form, and somehow "processed". The brain-based approaches seem to have their own laws and principles of operation that are somewhat independent of the mechanical functioning of the organism. But the conclusion of the previous section was that the brain approach and its more passive counterpart aren't really that different: they simply have (vastly) different levels of indirection.
Some of our oldest technologies, like mercury thermometers and hourglasses, rely on low-indirection feedback loops to be useful. And low-indirection "computation" is at the base of a new trend in robotics—possibly the most control-focused discipline of all—called morphological computation. That's a fancy name for an ancient idea: don't over-engineer a control mechanism when you can make your machine solve a problem by its shape alone. Here is a glimpse of that idea in action: a robot capable of walking down a slope using no power or sensors at all.
Seeing the Prophecies
In what way are all these low-indirection feedback loops "prophesy devices"? How are they "models of the outside world"?
I hinted at the answer in a previous post:
Those models are not the same as the physical things they are modeling. In fact, they are very different from most points of view—a network of a few thousand neurons looks and feels nothing like a door handle. That's alright. They don't need to be the same, as long as they behave similarly enough to produce reliable prophecies when and where you need them.
— From Embedded Prophesy Devices
To predict the future, a model only needs to capture the essential aspects of the external phenomena, and it needs to inform its parent system on how to steer towards its Water Lily for future benefit. The key is correlation: a good model produces an effect that "happens" to couple with the surrounding conditions to produce a specific, useful Water Lily in the future.
In most of these primitive cases, the "prediction" is as simple as
"The conditions an instant ago were X, so taking action Y will probably lead to <Water Lily> in the near future".
This is what the weather vane does ("The wind came from the north just now, so turning the arrow a little more to the north is likely to point in the direction of the wind—the Water Lily—in the next couple of seconds"). It's what the sea sponge does ("Dirt is clogging my pipes, so making a sneezing motion is likely to lead to my Water Lily of being a clean sponge"). And it's what E. coli does, as well ("I'm catching more nutrients than I was a few seconds ago, so keeping my current course will bring me closer to dinner").
I find this category of "low-indirection recursive phenomena" intriguing, because they have all the necessary ingredients without the mind-boggling complexity of brains and microchips. They allow you to focus on what's really fundamental in these processes, without having to worry about the intricacies of computer architectures and neuroscience.
All these examples teach us a major truth about how useful feedback works in biology and engineering: far from being idle predictions, of the kind a horse-racing gambler or a political pundit might make, these models directly contribute to the achievement of their own Water Lilies. They are all self-fulfilling prophecies.
If that sounds tautological (the predictions are the actions that realize the prediction), it's because these are loops. Of course they are both cause and effect. The meaning of "prediction" we're more familiar with is an extension of this into the realm of higher cognition. How that extension works is precisely what the next Plankton Valhalla essay will be about. ●
Cover image:
Photo by Tony Wright, Pexels